Introduction to Species Distribution Models (SDMs) and Their Challenges
Species distribution models (SDMs) are essential tools used to understand species distributions, ecological niches, and habitat suitability. Despite their broad applicability in ecology and conservation, SDMs often encounter significant challenges due to limitations in the available species occurrence data. These challenges include small sample sizes, positional uncertainty, and sampling biases, all of which can impact the accuracy and quality of the models‘ predictions. As a result, SDMs may sometimes fail to provide reliable insights, particularly for species with specific ecological requirements.
New Study on Data Limitations and Their Impact on SDMs
A recent study provides a comprehensive review of how these data limitations affect SDM performance. The authors examined previous research that focused on the impact of sample size, positional uncertainty, and sampling bias on SDM outputs. Based on these studies, they offer recommendations to improve the reliability of SDMs. Key recommendations include the need to critically assess species occurrence data before modeling, adjust sampling strategies to reduce bias, minimize positional errors, and apply methods like target-group background (TGB) to correct for sampling bias. TGB helps to align background data with the sampling bias present in the occurrence records, leading to more accurate model predictions.
Relationship Between Species Ecology and SDM Quality
Another important aspect highlighted by the study is the relationship between SDM quality and species ecology. Species with narrow geographic ranges or specialized ecological requirements are often more sensitive to data limitations compared to generalist species with wider distributions. Therefore, it is crucial to consider species-specific traits when collecting data and designing SDMs. For example, models predicting distributions of specialist species require more attention to detail in terms of data quality and sampling efforts, as even small errors can lead to inaccurate predictions.
The Importance of Addressing Data Quality for SDM Applications
The authors emphasize that addressing these challenges is vital for making SDMs more effective tools for various ecological applications, including species conservation, the study of invasive species, and predicting the impacts of climate change on biodiversity. As the interest in using SDMs continues to grow, the need to focus on data quality becomes increasingly important. Ensuring that species occurrence data are as accurate and representative as possible will help researchers and conservationists use SDMs more effectively in decision-making and policy planning.
Practical Guidelines for Improving SDM Reliability
This study provides valuable practical guidelines for researchers and conservation practitioners who use SDMs to assess species distributions. By carefully considering the limitations of species occurrence data and employing strategies to mitigate their effects, SDMs can become more robust and reliable tools for understanding and conserving biodiversity in a rapidly changing world.
See the complete article: https://nsojournals.onlinelibrary.wiley.com/doi/10.1111/ecog.07294
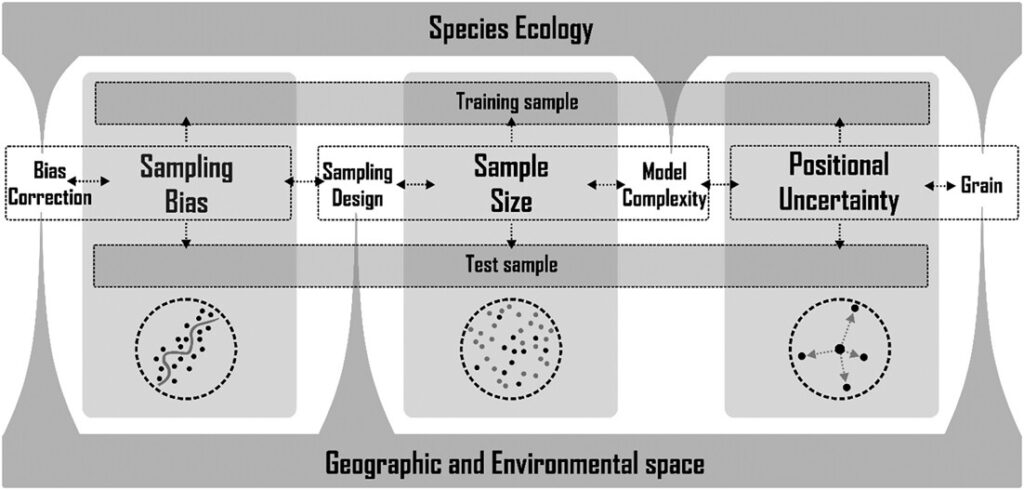
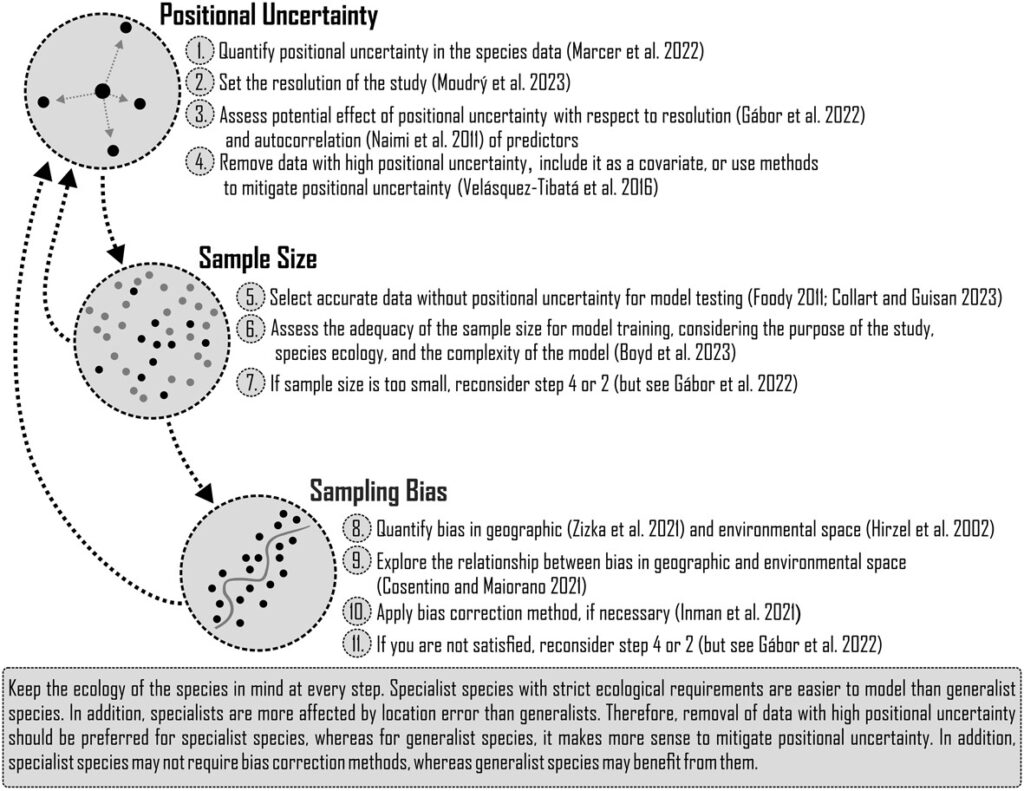