Author: Vitezslav Moudry
Published: January 11, 2025
From the very beginning of my doctoral studies, I have been obsessed with the quality and usability of spatial data. I have particularly focused on the importance of input data for correlative species distribution models (SDMs). SDMs are useful for tackling the gaps in our knowledge of species occurrence. However, despite their broad applicability, SDMs are limited by sample size, positional uncertainty, and sampling bias in species occurrence data. While numerous studies have evaluated the effects of these data limitations on SDM performance, including several from our department, a synthesis of their results was lacking. Now, thanks to EarthBridge and collaboration with several leading experts in this field, we have managed to summarize these shortcomings in a comprehensive review.
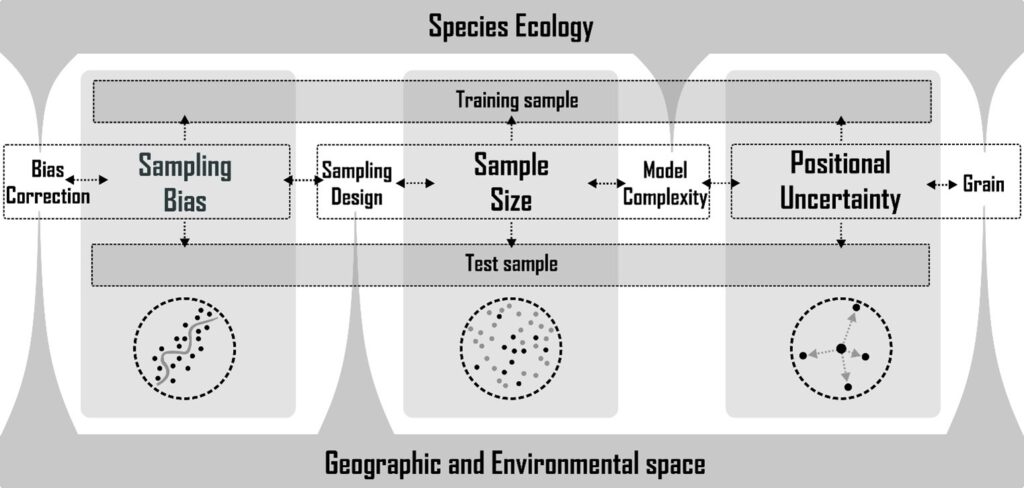
Sampling bias, sample size and positional uncertainty – the three characteristics of species occurrence data that affect the performance of your SDMs.
The extent to which modelling can replace fieldwork, in my opinion, remains an open question. On the one hand, models can help identify potential knowledge gaps and optimise the allocation of funds for field surveys (e.g. to pinpoint areas with a high potential for discovering unknown populations of species). On the other hand, the usability of predictions as guidelines for applications such as modelling species ranges, predicting responses to climate change, or planning conservation efforts is questionable. In any case, modelling species distributions must carefully consider the purpose of the study and the required sample size. The primary concern in the literature has been determining the minimum adequate sample size required to produce reliable models, as well as the extent to which additional resources should be invested to improve models by increasing sample size.
Furthermore, species occurrence data are always asociated with positional uncertainty, the magnitude of which (i.e., the difference between the actual and recorded location of a species) can range from a few meters (e.g., GNSS inaccuracies) to tens of kilometres (e.g., historical data). Under high positional uncertainty, SDMs using environmental layers at spatial resolutions finer than the magnitude of the positional uncertainty (e.g. environmental layers at a 10 m resolution and a 50 m positional uncertainty of species observations) can estimate erroneous species–environment relationships. The potential effect of positional uncertainty on SDMs performance is determined by several interacting factors, such as the resolution and spatial autocorrelation of predictors, as well as species ecology and site characteristics.
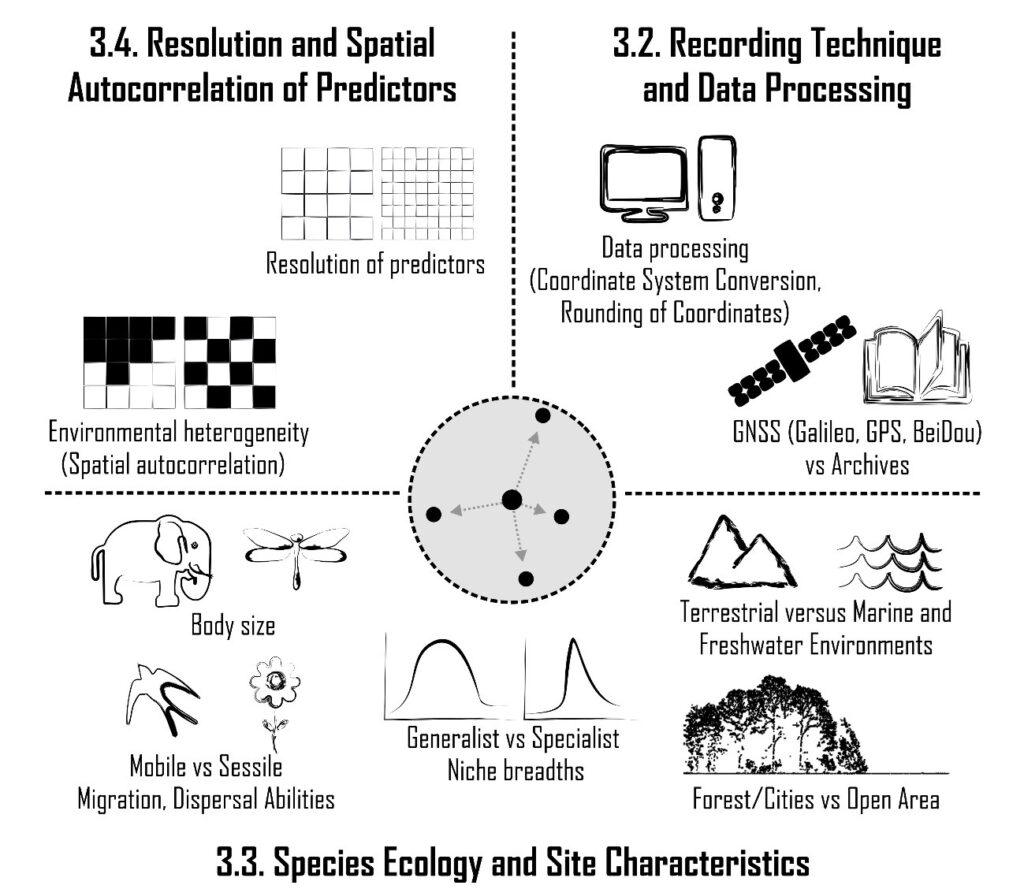
Three categories of factors driving positional uncertainty: the resolution and autocorrelation of predictors (e.g., micro- versus macroclimate data), recording techniques and data processing (e.g., GNSS accuracy) and species ecology and site characteristics (e.g., a lower accuracy for big mobile animals, limited GNSS accuracy under forest canopies or in cities)
A similar issue exists with sampling bias. Species observations often exhibit significant spatial bias, with many points clustered together and large gaps in between. Typically, positive sampling biases are reported towards easily accessible areas (e.g., proximity to roads and rivers), protected areas, more populated areas, and charismatic species. It is important to note, that the challenge in estimating species–environment relationships lies not only in the spatial bias within the geographic space where the bias originates but also in how this bias is reflected in the environmental space (i.e., the ecological niche). Although various methods have been proposed to compensate for sampling bias in both geographical and environmental space, their use must be approached with caution. Geographic and environmental spaces are communicating vessels, and so correcting one component (geographic or environmental) may have a detrimental effect on the other.
Importantly, our review extends beyond merely discussing the challenges associated with species occurrence data in SDMs. We provide practical recommendations for the critical assessment of species data intended for use in SDMs, summarized in the figure below.
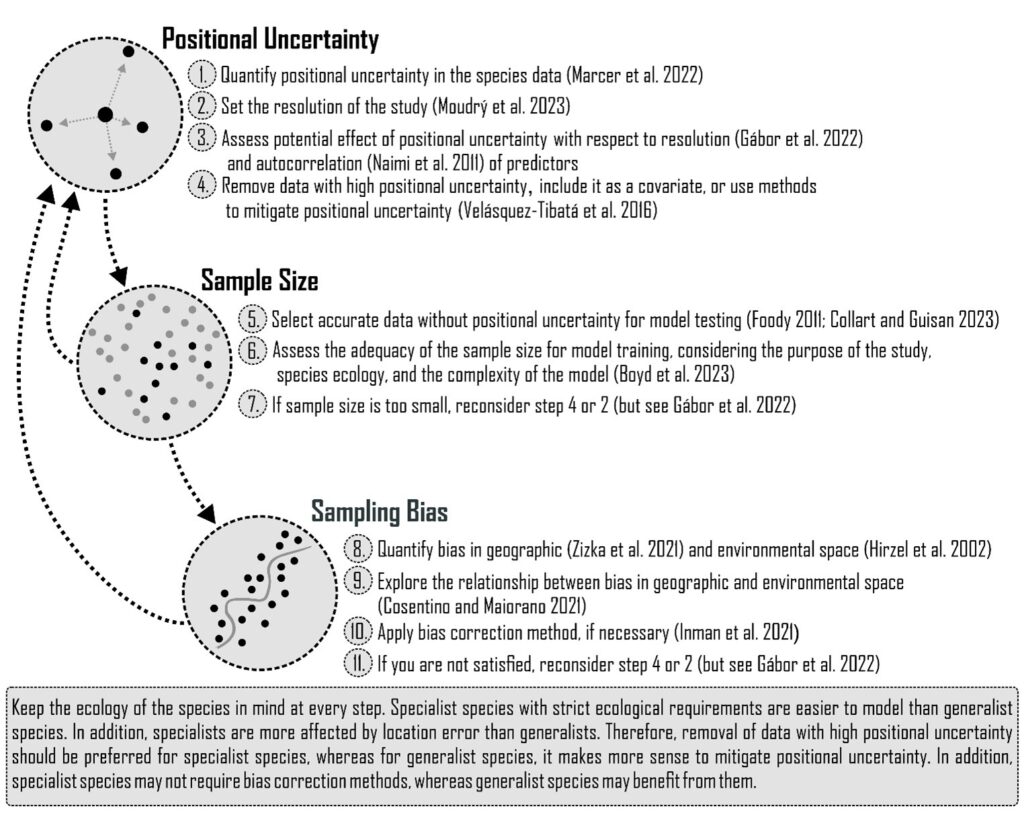
Workflow for a critical assessment of spatial data to be used in species distribution models (SDMs).
Without a comprehensive understanding of individual and combined effects of above mentioned issues, our ability to predict their influence on the quality of modelled species–environment associations remains largely uncertain, limiting the value of model outputs. If you feel like tackling some of the problems that still persist, we identified a few unanswered questions that are yet to be resolved. Read the whole review here in Ecography https://doi.org/10.1111/ecog.07294.