Research Article by Vítězslav Moudrý.
Introduction to GEDI Data Filtering
The Global Ecosystem Dynamics Investigation (GEDI) mission collects terrain and canopy height data using spaceborne lidar. While it provides invaluable forest and grassland height metrics, accurately using GEDI data requires filtering out noisy and low-quality observations affected by environmental and acquisition conditions. This study aims to refine data processing approaches for GEDI to maximize the usability of high-quality terrain and canopy height estimates in various environments, specifically focusing on Spain, California, and New Zealand.
Noise and Low-Quality Data Filtering
To enhance data accuracy, the study suggests starting with noise removal using criteria such as the „number of detected modes“ and „beam sensitivity.“ Observations with no detected modes were removed as noise, and in dense forests, a minimum sensitivity of 0.9 was necessary to retain accurate canopy data. However, in grasslands or areas with sparse vegetation, sensitivities as low as 0.5 were sufficient, as overly high sensitivity in grasslands often led to canopy overestimations. The combination of the quality flag (QF) and the difference from the TanDEM-X digital elevation model (DEM) was effective for filtering out low-quality data.
Additional Filtering Parameters
For further refinement, additional factors like the degrade flag, beam type, acquisition time (day vs. night), and terrain slope were evaluated. The degrade flag, specifically values 5, 50, and 85, was linked to lower data accuracy, and increased terrain slope resulted in terrain underestimations and canopy height overestimations due to multimodal waveform signals. However, time of acquisition and beam type had minimal effects on data accuracy, indicating their limited relevance in the data filtering process for temperate forests and grasslands.
Geolocation Error Mitigation and Future Recommendations
Positional adjustments of GEDI footprints improved terrain estimate accuracy but had limited impact on vegetation height accuracy, suggesting that geolocation correction is useful but not sufficient for canopy assessments. Future research should investigate GEDI data filtering across other biomes, as well as its influence on vertical canopy structure metrics. Combining filters like QF and TanDEM-X difference is recommended for balancing data quality and quantity, while sensitivity thresholds should be adjusted based on landcover to avoid unnecessary data loss in sparse vegetation areas.
See the whole article here: https://agupubs.onlinelibrary.wiley.com/doi/10.1029/2024EA003709
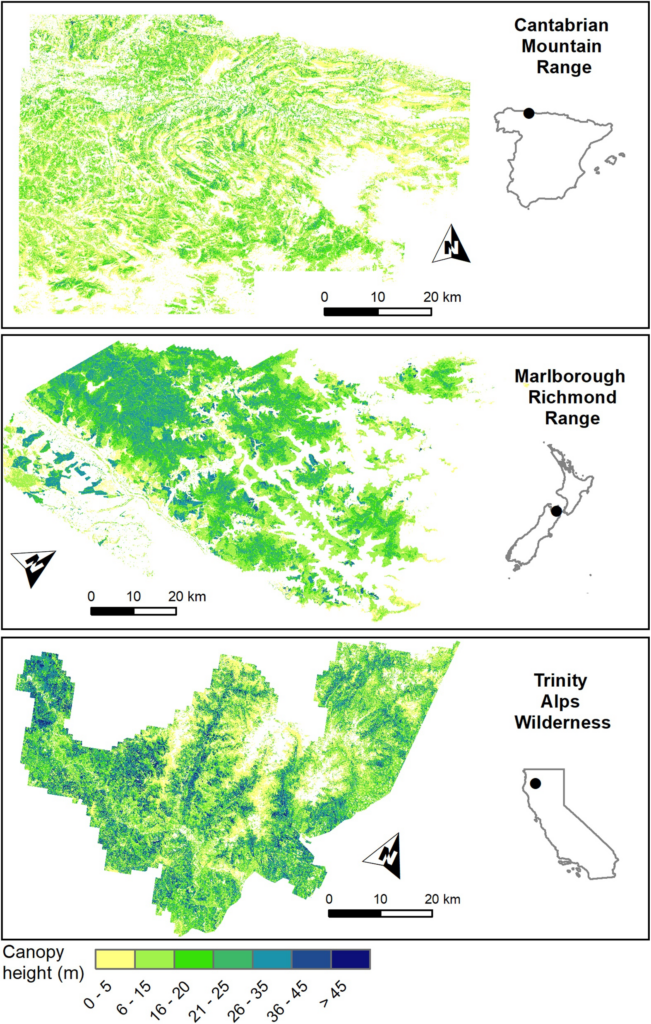
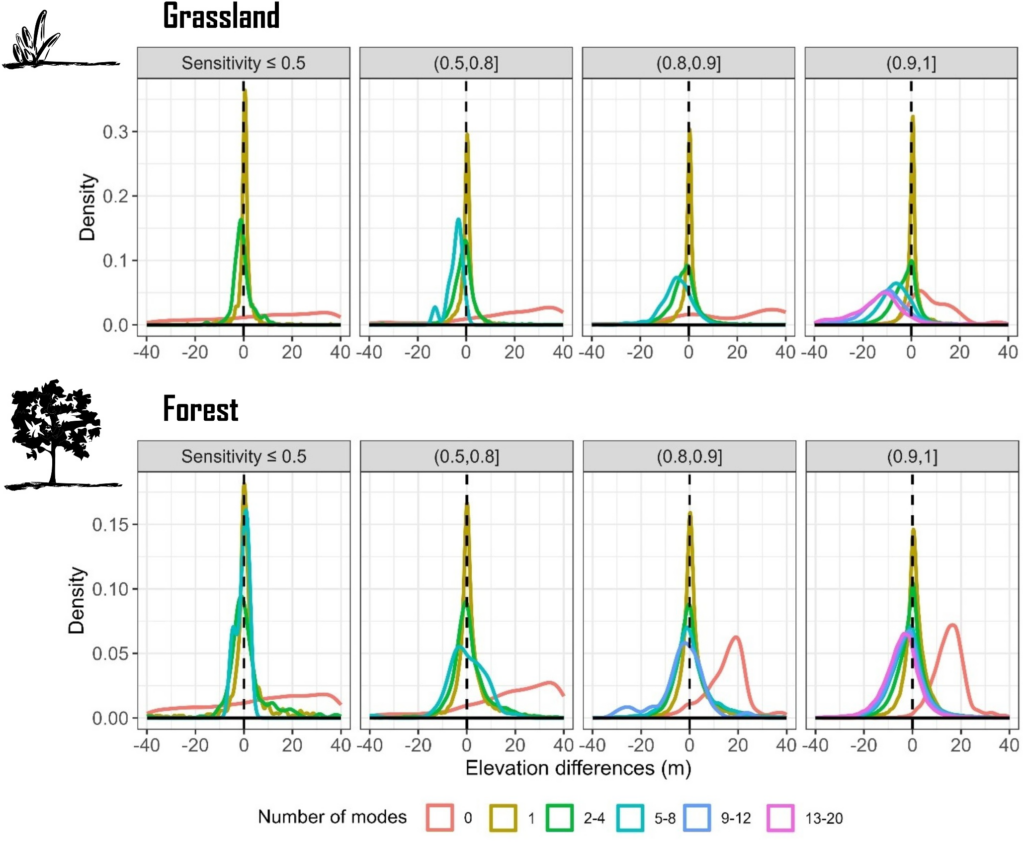